Insights
Warranty Data - Driving the Transformation of After-Sales Service-Part One
2024-02-01 11:38:18
Warranty Data - Driving the Transformation of After-Sales Service-Part One
Under the drive of the Industry 4.0 era, the basis of the corresponding transformation opportunities for after-sales service lies in data. Whether it's enterprises focusing on the development of connected products, the transformation of XaaS business model, digital transformation, installation excellent, resilient supply chains, omni-channel services, the deployment of digital twins as service enablers, or providing intelligent analytics, these all increase the importance of warranty data acquisition and analysis. Especially when facing the emergence of new technology products, geopolitical supply chain reorganization, and the shift of the customer experience economy, the overall industry strategy is gradually shifting from product-centric to customer-centric intelligent experiences[1].
IDC predicts that over 65% of businesses will invest in digitization to enhance product and service innovation within the next 5 years. This is especially true in the hope of product lifecycle management (PLM) and customer relationship management (CRM) collaboration, enhancing quality and customer satisfaction through product innovation. Whether it is remote monitoring, self-service, or asset proactive maintenance through intelligent systems, these all require effective filtering and analysis based on the foundation of warranty and service data acquisition to produce valuable information[2]. Warranty service and experience have become key drivers of value for customers, dealers, and operators. How to effectively collect warranty data and further analyze and manage it has become key to driving after-sales service transformation.
Data collection | ||||
The characteristics of different products will generate different warranty data. For example, the structure of iPhone warranty data is obviously different from that of large engine warranty data. The collection and application purposes of warranty data by different enterprises will also differ. Warranty data is essential for effective warranty management of existing products and new products. It can be basically divided into two major categories: warranty claim data and supplementary warranty data. | ||||
> | Warranty claim data: | |||
Data collected from the warranty claim process and repair service during the warranty period, which can be obtained from the after-sales warranty system. Service data, also known as warranty claim data, common warranty data includes actual after-sales warranty service activity records, including repair operations, failed parts, defect locations, failure causes, failure locations, and customer warranty claiming behavior and costs, etc. | ||||
> | Supplementary warranty data: | |||
Data obtained from within or outside the production organization, including data from design, research and development, production, market, etc., as well as external data from suppliers or other places, including shipping quantities, product specifications and bill of materials (BOM), supplier 0 information, etc. |
Through the collection and analysis of warranty claim data and supplementary warranty data, one can understand the failure patterns of products, and thereby establish reliability modeling, and even carry out effective management, analyze the difference with the expected reliability and make improvements. |
Table 1 - Different data sources and purposes in different scenarios Table 1 lists the sources and purposes of related warranty data collection under various scenarios, taking the equipment manufacturing and commodity sales industries as examples. Data not only exists in the external customer use process, but also hides in the internal management process of the enterprise, such as machine management in manufacturing, quality control of materials, and control of peripheral support asset, etc. Many warranty data are messy and unstructured, which is the main obstacle for enterprises to do warranty data analysis. Systematic and continuous data collection and filtering of anomalies to avoid causing subsequent analysis errors is an important link in establishing subsequent application and analysis data. This is often the key reason why enterprises cannot effectively provide warranty data analysis. |
Data Structure | ||||
Data quality is highly related to subsequent warranty statistical analysis and reliability determination. Before applying data description and analysis methods, one must carefully check the correctness of the data and whether it is in line with the principles of research objectives. The purpose of preliminary data inspection is to verify the data source, whether it includes designated variables, measurement units, and at the same time delete or correct obvious errors, and compile a complete data structure. |
> | Data Check: | |||
For abnormal values or results, it's essential to check for missing data. This is particularly crucial in the analysis of warranty claim data processing. Service data is often messy and prone to errors due to differences in sources and systems. The key to collecting data and performing the right analysis lies in understanding the data structure. For warranty claims or other supplementary data, ensure the dataset is updated and complete. The warranty data collected by the enterprise mainly includes structure one, which contains the sales date and failure time of the product; and structure III, which is the total number of failures within discrete time intervals. | ||||
> | Structure I Data: | |||
Mainly warranty records by product numbers or specific serial numbers which is majorly applied in the repair history of high-priced products to understand the number and status of product failures in order to estimate Mean Time to Failure. Table 2 is an example of basic Structure I Data, in which failure time can also be other variables, such as kilometers, cycles of use, etc. The focus is on recording the duration of product use before failure, which is most suitable for estimating failure distribution, reliability, hazard function and related functions. |
Table 2 - Table of Structure I Data [3] | ||||
> | Structure III Data: | |||
As shown in Table 3, the cycle of failures for individual products are recorded by product sales or production time to effectively compare the quality changes of different batches of products on the customer end. Such structure can be applied to mass product warranty data tracking, calculation of basis for Warranty Claim Rate (WCR) and quality improvement; meanwhile, the duration of product use prior to failure can be calculated. To simplify the calculation, it is assumed that the interval between sales and production time is not large, and the relationship between product production time and work time can be understood. |
Table 3 - Table of Structure III Data[3] | ||||
The appropriate analysis method depends on the analysis purpose and data structure. After confirming the data structure, the first step is to define the interval for data collection and let the system automatically collect data for analysis through the set period. Warranty operations will also vary depending on the customer's warranty contracts, such as return for repair, exchange, or proportional refund. These will affect the assumptions of warranty data collection and reliability modeling. |
Author
Dr. Albert Liao
Founder and CEO of Wareconn Technology Services (wareconn.com),
Author of “Warranty Chain Management - Digitalization and Sustainability”, Springer
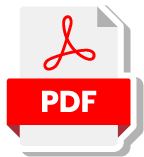
Reference
- Stephane Scouchet, Vinodkumar Ramachandran, 2023, Future of smart industrials – KPMG Connected Enterprise for engineered products aftermarket and field service, KPMG internationals.
- Aly Pinder, 2023, Worldwide Service Life-Cycle Management Platforms 2023-2024 Vendor Assessment, IDC MarketScape, IDC #US49989623.
- Wallace R. Blischke, M. Rezaul Karim, D. N. Prabhakar Murthy, “ Warranty Data Collection and Analysis”, Springer, 2011.
- Douglas Thomas, Brian Weiss, (2021), Maintenance Costs and Advanced Maintenance Techniques in Manufacturing Machinery: Survey and Analysis, International Journal of Prognostics and Health Management.
- “Twentieth Annual Product Warranty Report”, warrantyweek.com, 2023
- Albert Liao, (2022),Warranty Chain Management - Digitalization and Sustainability, Springer.
- Aly Pinder, etc, 2022, Worldwide Manufacturing Product and Service Innovation 2023 Predictions, IDC Future Scape, IDC #US48627622.
- “Powering warranty reinvention, How electronics companies leverage new technologies to improve warranty management”, IBM Institute for Business Value, 2017.